The healthcare industry has been producing massive amounts of data at an unprecedented rate in recent years. Electronic health records, medical images, sensor data, and other health-related information are examples of this data. The collection, processing, and analysis of these large and complex datasets to extract valuable insights that can inform healthcare decisions and improve patient outcomes is referred to as big data.
Because of the potential to transform the way healthcare is delivered and managed, the use of big data in medical care has grown in importance. Healthcare providers can identify patterns and trends in patient health, improve diagnosis and treatment, and reduce costs by analysing massive amounts of data. Furthermore, big data can help clinical research by providing real-world data and evidence to develop and test new treatments.
We will look at the characteristics of big data in medical care, the challenges of processing and analysing medical care data, and the applications of big data in clinical research and medical care delivery in the sections that follow. We will also go over the ethical implications of using patient data in medical care research.
How is big data used in health?
Big data is used in various ways in the medical care industry to improve patient outcomes and enhance the delivery of healthcare services. Some of the key applications of big data in medical care include:
Identifying patterns and trends :
By analyzing large and complex healthcare datasets, big data can help medical care providers identify patterns and trends in patient health that may not be apparent through traditional diagnostic methods. This can aid in early detection and prevention of diseases, improve diagnosis accuracy, and inform treatment decisions.
Improving healthcare outcomes and patient safety:
Big data can help health providers deliver more personalized and effective care by identifying the most appropriate treatment options for individual patients based on their unique medical history, genetic makeup, and other factors. This can lead to better patient outcomes and reduced healthcare costs. Moreover, big data can help identify potential safety risks and enable healthcare providers to take proactive measures to mitigate them.
Enhancing healthcare delivery and resource utilization:
Big data can help health providers optimize resource utilization by identifying areas where improvements can be made in efficiency and cost-effectiveness. For example, it can help hospitals optimize staff schedules, reduce readmission rates, and manage inventories more effectively.
Supporting research and development of new treatments and therapies:
Big data can support clinical research by providing real-world data and evidence that can be used to develop and validate new treatments and therapies. It can also help identify potential areas of research and provide insights into the effectiveness of existing treatments.
To summarise, big data has the potential to transform the healthcare industry by providing valuable insights that can inform healthcare decisions, improve patient outcomes, and improve healthcare service delivery.
What are the main characteristics of big data in healthcare?
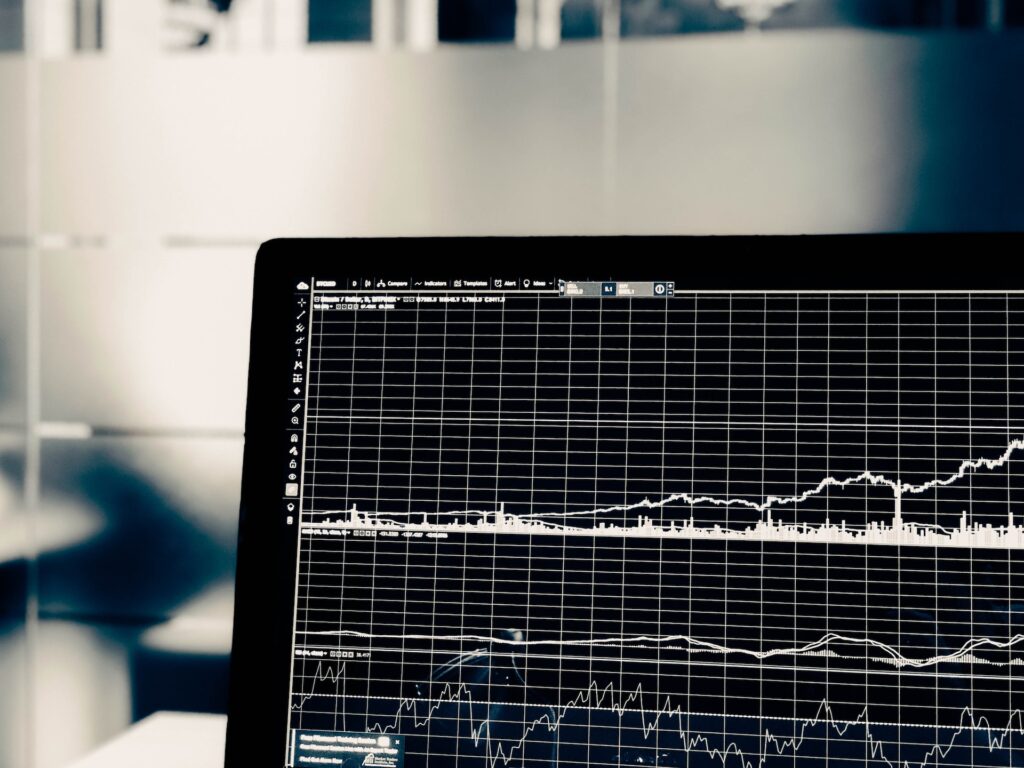
Big data in medical care is characterized by four main attributes: volume, variety, velocity, and veracity.
Volume refers to the sheer amount of data generated in the healthcare industry, which includes electronic health records, medical images, sensor data, and other health-related information. The volume of healthcare data is increasing exponentially and is expected to reach 2,314 exabytes by 2020.
Variety refers to the different types of data generated in the healthcare industry, including structured and unstructured data. Structured data is data that can be easily organized and analyzed, such as electronic health records. Unstructured data, on the other hand, is data that is more difficult to analyze, such as medical images and text documents.
Velocity refers to the speed at which healthcare data is generated, collected, and analyzed. Healthcare data is generated in real-time and requires rapid analysis to inform healthcare decisions and improve patient outcomes.
Veracity refers to the accuracy and reliability of healthcare data. Healthcare data is often generated from multiple sources and may contain errors, inconsistencies, and biases. Ensuring the veracity of healthcare data is essential to making informed decisions and achieving accurate results.
Large healthcare datasets, on the other hand, can be difficult to manage and process. Healthcare data is difficult to store, manage, and analyse due to its sheer volume and complexity. Healthcare data is frequently stored in disparate formats and systems, making integration and analysis difficult. Furthermore, data quality and standardisation are critical to ensuring healthcare data accuracy and consistency.
Also read : Gene Editing: Advancements, Techniques, Applications, Ethics, and Social Concerns
Big data processing
Big data processing involves the use of technologies and techniques to store, manage, and analyze large healthcare datasets. In healthcare, big data processing is essential to making informed decisions, improving patient outcomes, and enhancing the delivery of healthcare services.
Technologies for storing and managing large healthcare datasets:
One of the primary challenges of big data processing in healthcare is storing and managing large and complex datasets. Technologies such as Hadoop, NoSQL, and Apache Spark are commonly used to store and manage healthcare data. These technologies are designed to handle large volumes of data and provide scalability, reliability, and fault tolerance.
Data processing and cleaning techniques:
Once healthcare data is stored, it must be processed and cleaned to ensure its accuracy and consistency. Data processing techniques such as data mining, machine learning, and natural language processing can be used to extract valuable insights from healthcare data. Data cleaning techniques such as data deduplication, data normalization, and data validation are used to remove errors and inconsistencies from healthcare data.
Data integration and interoperability:
Healthcare data is often stored in different formats and systems, making it difficult to integrate and analyze. Data integration techniques such as extract, transform, and load (ETL) can be used to integrate healthcare data from different sources. Interoperability standards such as Fast Healthcare Interoperability Resources (FHIR) can also be used to ensure that healthcare data is exchanged seamlessly between different healthcare systems and applications.
Big data analytics in healthcare
Healthcare providers use Big data analytics to make data driven decisions, improve patient outcomes and enhance the delivery of health care services. It also uses the advanced analytical techniques to get insights and knowledge form large healthcare datasets
Descriptive, predictive, and prescriptive analytics:
There are three types of big data analytics in healthcare: descriptive, predictive, and prescriptive. Descriptive analytics provides information about what happened in the past. Predictive analytics makes forecasts for future events based on historical data.Then best course of action is recommended by prescriptive analytics based on the predictions of the predictive analytics
Machine learning and artificial intelligence :
They are critical components of big data analytics. Machine learning algorithms can be used to analyse large healthcare datasets and identify patterns and trends that traditional data analysis methods may miss. Artificial intelligence can also be used to create predictive models for disease diagnosis and treatment.
Data visualisation and dashboarding:
Charts, graphs, and dashboards are examples of data visualisation techniques that can be used to present healthcare data in a more accessible and understandable format. These techniques allow healthcare providers to identify patterns and trends in healthcare data quickly and make data-driven decisions.
The development of predictive models for disease diagnosis and treatment
One of the key applications of big data analytics in healthcare is the development of predictive models for disease diagnosis and treatment. Predictive modeling uses historical healthcare data to develop models that can be used to predict future healthcare outcomes. These models can be used to inform clinical decision-making, optimize resource allocation, and improve patient outcomes.
Applications of predictive analytics in healthcare:
Predictive analytics is used in healthcare for a range of applications, including disease diagnosis and treatment, patient risk assessment, and resource allocation. Predictive models can be used to identify patients at risk of developing a particular disease or condition, predict the likelihood of a patient responding to a particular treatment, and forecast the expected length of hospital stay.
Examples of successful predictive models in disease diagnosis and treatment:
One example of a successful predictive model in disease diagnosis and treatment is the use of machine learning algorithms to predict patient outcomes in sepsis. Another example is the development of a predictive model for early detection of breast cancer using mammography data.
Challenges and limitations of predictive modeling in healthcare:
Predictive modeling in healthcare faces several challenges and limitations, including data quality issues, data privacy concerns, and the need for domain expertise. It can also be difficult to implement predictive models in clinical practice, as they may not always align with clinical decision-making processes or the needs of individual patients.
Use of big data in clinical research
Big data is increasingly being used in clinical research to inform trial design and execution, generate real-world evidence, and enhance regulatory decision-making.
Role of big data in clinical trial design and execution:
Big data can be used to inform clinical trial design by identifying eligible patient populations, selecting appropriate endpoints, and optimizing trial logistics. It can also be used to monitor trial progress and identify potential safety issues.
Opportunities for real-world data and evidence generation:
Big data provides opportunities for real-world data and evidence generation by leveraging data from electronic health records, claims data, and other sources. Real-world evidence can be used to support regulatory decision-making, supplement clinical trial data, and inform healthcare policy decisions.
Regulatory considerations for big data use in clinical research:
Using big data in clinical research raises several regulatory concerns, including data privacy, security, and informed consent. Regulators are also investigating the use of real-world evidence in regulatory decision-making and developing guidelines for big data use in clinical research.
The ethical considerations surrounding the use of patient data in healthcare research
The use of patient data in healthcare research raises several ethical considerations related to data privacy, informed consent, data sharing, and the balance of benefits and risks.
Data privacy and confidentiality:
Patient data must be treated with the utmost respect for privacy and confidentiality. Researchers must ensure that patient data is de-identified or anonymized to protect patient privacy and comply with data protection regulations.
Informed consent and patient autonomy:
Patients must provide informed consent for the use of their data in research, and they must have the option to withdraw their consent at any time. Researchers must ensure that patients are fully informed about the purpose of the research, the risks and benefits of participation, and any potential conflicts of interest.
Data sharing and ownership:
Data sharing is an important aspect of healthcare research, but it also raises concerns about data ownership and control. Researchers must ensure that data is shared in a responsible and transparent manner and that patients are informed about how their data will be used.
Risks and benefits of using patient data in research:
Usage of patient data in research can lead to substantial benefits for both patients and society, like the development of new therapies and treatments. But, it raises concerns about data privacy, confidentiality, and potential misuse. Researchers must ensure that the advantages outweigh the disadvantages while using patient data in research
In conclusion, big data has the potential to revolutionize healthcare by improving patient outcomes, enhancing healthcare delivery, and supporting research and development of new treatments and therapies. However, the use of big data in healthcare also raises several challenges and ethical considerations that must be addressed to ensure that patient privacy and autonomy are respected and that the benefits of using patient data in research outweigh the potential risks.
Conclusion:
In conclusion, big data has the potential to transform healthcare by improving patient outcomes, enhancing healthcare delivery, and supporting research and development of new treatments and therapies. Big data analytics can identify patterns and trends in healthcare data, inform clinical decision-making, and optimize healthcare resource utilization.
However, the use of big data in healthcare also presents several challenges, including data privacy and security, data quality and standardization, and the need for advanced data processing and analytical techniques. Ethical considerations related to patient privacy, informed consent, and data sharing must also be addressed to ensure that patient rights and autonomy are respected.
Looking to the future, the use of big data in healthcare is expected to continue to grow, driven by advances in data collection and processing technologies, the increasing availability of real-world data, and the adoption of artificial intelligence and machine learning techniques. However, challenges related to data governance, interoperability, and data sharing must be addressed to ensure that the full potential of big data in healthcare is realized.
In summary, big data has the potential to revolutionize healthcare, and its importance and potential should not be overlooked. By addressing the challenges and ethical considerations associated with the use of big data in healthcare, we can ensure that patients benefit from the full potential of this powerful technology.
You’re so awesome! I don’t believe I have read a single thing like that before. So great to find someone with some original thoughts on this topic. Really.. thank you for starting this up. This website is something that is needed on the internet, someone with a little originality!
Fantastic site A lot of helpful info here Im sending it to some buddies ans additionally sharing in delicious And naturally thanks on your sweat
I was recommended this website by my cousin I am not sure whether this post is written by him as nobody else know such detailed about my difficulty You are wonderful Thanks
Wow wonderful blog layout How long have you been blogging for you make blogging look easy The overall look of your site is great as well as the content
I do not even know how I ended up here but I thought this post was great I do not know who you are but certainly youre going to a famous blogger if you are not already Cheers